- Journal List
- PLoS One
- v.8(2); 2013
- PMC3562340

Detection of Error Related Neuronal Responses Recorded by Electrocorticography in Humans during Continuous Movements
Conceived and designed the experiments: TM TB CM. Performed the experiments: TM TB CM. Analyzed the data: TM TB. Wrote the paper: TM TB ASB AA CM.
Abstract
Background
Brain-machine interfaces (BMIs) can translate the neuronal activity underlying a user’s movement intention into movements of an artificial effector. In spite of continuous improvements, errors in movement decoding are still a major problem of current BMI systems. If the difference between the decoded and intended movements becomes noticeable, it may lead to an execution error. Outcome errors, where subjects fail to reach a certain movement goal, are also present during online BMI operation. Detecting such errors can be beneficial for BMI operation: (i) errors can be corrected online after being detected and (ii) adaptive BMI decoding algorithm can be updated to make fewer errors in the future.
Methodology/Principal Findings
Here, we show that error events can be detected from human electrocorticography (ECoG) during a continuous task with high precision, given a temporal tolerance of 300–400 milliseconds. We quantified the error detection accuracy and showed that, using only a small subset of 2×2 ECoG electrodes, 82% of detection information for outcome error and 74% of detection information for execution error available from all ECoG electrodes could be retained.
Conclusions/Significance
The error detection method presented here could be used to correct errors made during BMI operation or to adapt a BMI algorithm to make fewer errors in the future. Furthermore, our results indicate that smaller ECoG implant could be used for error detection. Reducing the size of an ECoG electrode implant used for BMI decoding and error detection could significantly reduce the medical risk of implantation.
Introduction
Even though the control of prosthetic devices using brain-machine interfaces (BMIs) has highly improved during the last several years
The following popper user interface control may not be accessible. Tab to the next button to revert the control to an accessible version.
Destroy user interface control[1]–The following popper user interface control may not be accessible. Tab to the next button to revert the control to an accessible version.
Destroy user interface control[3], such devices are still prone to decoding errors. Decoding errors can elicit error related neuronal responses (ERNRs). Detecting these errors can be beneficial for the BMI performance. If detected, errors can be subsequently corrected, recognizing that a certain effector movement was not intended. This strategy has already been implemented in on-line BMI studiesThe following popper user interface control may not be accessible. Tab to the next button to revert the control to an accessible version.
Destroy user interface control[4]–The following popper user interface control may not be accessible. Tab to the next button to revert the control to an accessible version.
Destroy user interface control[7], but only in externally paced BMIs. However, many powerful BMIs are used to continuously decode and control the movements of an effector. Most prominent examples are the BMI control of a prosthetic armThe following popper user interface control may not be accessible. Tab to the next button to revert the control to an accessible version.
Destroy user interface control[1],The following popper user interface control may not be accessible. Tab to the next button to revert the control to an accessible version.
Destroy user interface control[8]–The following popper user interface control may not be accessible. Tab to the next button to revert the control to an accessible version.
Destroy user interface control[10] and the brain control of a computer cursorThe following popper user interface control may not be accessible. Tab to the next button to revert the control to an accessible version.
Destroy user interface control[3],The following popper user interface control may not be accessible. Tab to the next button to revert the control to an accessible version.
Destroy user interface control[9],The following popper user interface control may not be accessible. Tab to the next button to revert the control to an accessible version.
Destroy user interface control[11].Error detection can also be used to modify the decoding algorithm to make fewer decoding errors in the future. This approach is especially suitable for BMIs decoding continuous movements, since subjects correct for movement discrepancy by producing corrective movements, thereby making subsequent error correction obsolete. However, the feasibility of this strategy has so far been demonstrated only by computer simulations
The following popper user interface control may not be accessible. Tab to the next button to revert the control to an accessible version.
Destroy user interface control[12],The following popper user interface control may not be accessible. Tab to the next button to revert the control to an accessible version.
Destroy user interface control[13].To apply error detection to continuous BMI control, it is necessary to show that ERNR are indeed elicited during such tasks. A number of studies investigated neuroal responses to errors during continuous movement tasks, identifying neuronal activity related to three different error types: (i) target error
The following popper user interface control may not be accessible. Tab to the next button to revert the control to an accessible version.
Destroy user interface control[14],The following popper user interface control may not be accessible. Tab to the next button to revert the control to an accessible version.
Destroy user interface control[15], event where the task environment goes through unexpected changes such as target jumps, (ii) execution errorThe following popper user interface control may not be accessible. Tab to the next button to revert the control to an accessible version.
Destroy user interface control[14],The following popper user interface control may not be accessible. Tab to the next button to revert the control to an accessible version.
Destroy user interface control[16], event where the ongoing motor commands result in an unexpected movement, and (iii) outcome errorThe following popper user interface control may not be accessible. Tab to the next button to revert the control to an accessible version.
Destroy user interface control[16]–The following popper user interface control may not be accessible. Tab to the next button to revert the control to an accessible version.
Destroy user interface control[19], event where the desired goal of a movement is not achieved. Outcome and execution errors are of special interest for BMI application, since both error events can occur during online BMI control. For example, an execution error can occur when the decoding algorithm decodes incorrect movements, thereby moving the cursor or the prosthesis in an undesirable direction (Figure 1). If the difference of intended and executed movements is large enough, it can evoke an execution ERNR. If the incorrectly decoded movement causes the effector to reach an unintended goal or perform an unintended function, this can elicit an outcome ERNR (Figure 1).In recent years, electrocorticography (ECoG) emerged as a possible alternative to intracortical recordings as a recording technique that can be used for a continuous BMI
The following popper user interface control may not be accessible. Tab to the next button to revert the control to an accessible version.
Destroy user interface control[20]–The following popper user interface control may not be accessible. Tab to the next button to revert the control to an accessible version.
Destroy user interface control[22]. Continuous BMI controlling two degrees of freedom has already been realized using ECoGThe following popper user interface control may not be accessible. Tab to the next button to revert the control to an accessible version.
Destroy user interface control[23]. Other recent studies showed that many other movement primitives can be decoded offline from ECoG signals, such as 7 degrees of freedom of arm movementsThe following popper user interface control may not be accessible. Tab to the next button to revert the control to an accessible version.
Destroy user interface control[24], individual finger movementsThe following popper user interface control may not be accessible. Tab to the next button to revert the control to an accessible version.
Destroy user interface control[25] and natural graspsThe following popper user interface control may not be accessible. Tab to the next button to revert the control to an accessible version.
Destroy user interface control[26]. Therefore, ECoG is a suitable platform for implementing a continuous BMI.To use error signals in a ECoG based BMI, one needs to show that ERNRs can be detected from the ECoG signal with sufficient reliability. A recent study by Milekovic et al.
The following popper user interface control may not be accessible. Tab to the next button to revert the control to an accessible version.
Destroy user interface control[16] showed that both outcome and execution ERNR are present in human ECoG recordings during a continuous task. Here, we showed that the times of the events that elicited these ERNR can be detected with high accuracy.Methods
Experimental task, recording techniques and properties of the recoded data are described in detail in Milekovic et al.
The following popper user interface control may not be accessible. Tab to the next button to revert the control to an accessible version.
Destroy user interface control[16]. Here we provide only a short description.Task
Subjects (S1–S4) played a simple video game in which they controlled a spaceship with a small analogue joystick on a gamepad (Logitech® Rumblepad™ 2, Logitech Europe S.A., Morges, Switzerland) in the horizontal dimension (left-right; Figure 2a; Supplementary movie 1). The task was to evade blocks dropping from the top of the screen at a constant speed. The game was challenging enough so that the spaceship occasionally collided with a block (collision event, Figure 2b). At random moments, the spaceship moved in the direction opposite to the joystick movement for the duration of 500 ms (movement mismatch event, Figure 2c). Points were awarded for moving the spaceship, and subjects were instructed to gather as many points as possible. Subjects started the game with 20 “lives”. Each time the spaceship collided with a block, the number of “lives” was reduced by one. When the number of “lives” reached 0, the game, together with the recording session, ended. Recording sessions lasted between 5 and 24 minutes. We identified the neuronal responses to collision and mismatch events that were not mixed with neuronal responses to other events by defining a subgroup of “clean” outcome and “clean” mismatch events, consisting of events at least 2 seconds away from any other event of any kind. The total number of events recorded for each of the subjects is given in Table 1.
To earn more points, subjects had to try to stay in the game as long as possible. Therefore, collision events presented a clear disadvantage in reaching the goal of the game. Thus, collision events reflected outcome errors. During the movement mismatch event, there was a clear discrepancy between the intended movement and the movement performed by the spaceship. Thus, movement mismatch events reflected execution errors.
In our previous study
The following popper user interface control may not be accessible. Tab to the next button to revert the control to an accessible version.
Destroy user interface control[16] we demonstrated that the ECoG signals we used for detection in the present study reflect ERNRs and are not movement related, nor related to visual stimuli or to surprise effects.Subjects and Recordings
Four subjects (3 male, 1 female) suffering from intractable pharmaco-resistant epilepsy voluntarily participated in the study after having given their informed consent. The study was approved by the Ethics Committee of the University Medical Center, Freiburg, Germany.
For pre-neurosurgical epilepsy diagnosis, the subjects were implanted with an 8×8 grid of subdural surface electrodes covering parts of the primary and pre-motor cortex (Figure 3). Additional subdural surface and deep brain electrodes were implanted for subjects S1, S2 and S3
The following popper user interface control may not be accessible. Tab to the next button to revert the control to an accessible version.
Destroy user interface control[16]. For all subjects, signals from 22 electrodes of EEG, two to four electrooculogram (EOG) electrodes, electrocardiogram (ECG) and electromyogram (EMG) were recorded simultaneously with the recordings made from the subdural and deep brain electrodes. Here, we analyzed the recordings from the 8×8 grid of subdural electrodes only.Recordings from all electrodes were digitized at 256 Hz sampling rate for S1 and S2 and at 1024 Hz sampling rate for S3 and S4. No analogue filters were used during the data acquisition. Power line frequency was 50 Hz. Data analysis presented here was performed after the experiment using the MATLAB software package (MATLAB version 7.4–7.11, Natick, Massachusetts: The MathWorks Inc., 2007–2011).
Measures of Detection Accuracy
Consider a process where a subject is actively observing a scene and, when a given stimulus appears, a neuronal response is elicited. Assume that neuronal activity is continuously recorded and a detection algorithm is continuously evaluating whether a stimulus appeared, given the neuronal activity. The efficiency of the detector can then be measured by comparing two point processes: the set of time points when the stimulus was presented and the set of time points when the detector detected the stimulus from the neuronal recordings.
Due to the internal processes in the brain and other sources of noise, even a perfect decoder will have a temporal noise in the detected times of events. On the other hand, detected events will still be useful, even if the times of detections are not perfectly aligned to the times of the events. For some applications, high temporal precision is not necessary. We describe this requirement on our detector as temporal tolerance.
If we tolerate the detected events within a time Δt from the real events, then any detection within this time window will be counted as a true positive detection. Every event window in which there are no detected events will be counted as false negative detection. For measuring the detection accuracy, we would also need to know the ability of the detector at predicting non-events. To obtain a fair estimate of such ability, the area between the event time windows has to be divided in windows of the same size, 2Δt. Every non-event time window in which there are no detected events will be counted as a true negative detection and every non-event time window in which there is a detected event will be counted as false positive detection.
Sensitivity and specificity of a detector
Accuracy of a detector can be described by measuring how well it performs in two different tasks: (i) detecting events when events are present and (ii) not detecting events when events are not present. One way to describe the first property is by measuring the sensitivity of the detector by calculating the true positive rate (TPR)
The following popper user interface control may not be accessible. Tab to the next button to revert the control to an accessible version.
Destroy user interface control[27] as a number of true positive detections (NTP) divided by the total number of real events. Since the total number of real events is given by the sum of true positive detections and false negative detections (NFN), the true positive rate is given by: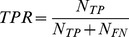
The second property can be described by measuring the specificity of the decoder by calculating the false positive rate (FPR)
The following popper user interface control may not be accessible. Tab to the next button to revert the control to an accessible version.
Destroy user interface control[27] as the ratio of false positive detections (NFP) divided by the number of all detections. Since the total number of all detections is given by the sum of true positive detections and false positive detections (NFP), the false positive rate is given by: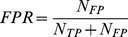
FPR definition used here should not be confused with the alternative definition of the false positive rate (FPRALT)
The following popper user interface control may not be accessible. Tab to the next button to revert the control to an accessible version.
Destroy user interface control[28]: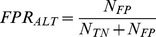
A disadvantage of measuring the detection accuracy by TPR and FPR is that one cannot directly compare two different detectors when both TPR and FPR of one detector are higher than the TPR and FPR of the other detector. Therefore, a metric incorporating both sensitivity and specificity of the detector is needed. One such metric is the mutual information.
Mutual information of a detector
One way to measure the performance of a detector is to calculate the mutual information
The following popper user interface control may not be accessible. Tab to the next button to revert the control to an accessible version.
Destroy user interface control[29] between a dataset containing times of real events and a dataset containing times of detected events. The mutual information is given by:
where X and Y are the sets of all possible states of the real and detected event datasets and x and y are specific states from those sets, p(x) and p(y) are the probabilities of specific states and p(x,y) is the joint probability that states x and y occur jointly. In our case, the set of real event states consists of “real event” (re) and “real non-event” (rne), while the set of detected events consists of “detected event” (de) and “detected non-event” (dne). Joint and marginal probabilities used to calculate the mutual information are given by:
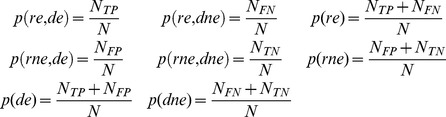
Given a certain dataset of real event times and certain tolerance, the maximum value of the mutual information is obtained when detected event times perfectly match real event times. This value is identical to the entropy of real event times H(X):

To compare the mutual information over different tolerances, we calculated the normalized mutual information, CYX
The following popper user interface control may not be accessible. Tab to the next button to revert the control to an accessible version.
Destroy user interface control[30]: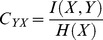
Calculating mutual information and entropy from a recorded dataset will give a good estimate of their true values, as long as the calculated probabilities are good estimates of the real probabilities. However, recorded datasets have a finite length, which will make the estimated probabilities fluctuate around their real values. Using the estimated probabilities to calculate the mutual information and entropy leads to a bias in the estimation
The following popper user interface control may not be accessible. Tab to the next button to revert the control to an accessible version.
Destroy user interface control[31]. To remove the bias of the mutual information, we used first and second order terms of the mutual information bias expansion derived in the study by Treves and PanzeriThe following popper user interface control may not be accessible. Tab to the next button to revert the control to an accessible version.
Destroy user interface control[31]:


where IN(X,Y) is the mutual information estimated from a dataset of length N. Here, the values of joint and marginal distributions have also been estimated from the same dataset. To remove the bias of the entropy, we used first and second order terms of the entropy bias expansion derived in the study by Victor
The following popper user interface control may not be accessible. Tab to the next button to revert the control to an accessible version.
Destroy user interface control[32]:


Thus, the bias corrected value of the normalized mutual information was calculated as:
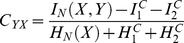
Experimental Data Analysis
Preprocessing
Common-average referencing for grid electrodes was performed using all grid electrodes that showed no artefacts (one electrode for both S3 and S4 had to be excluded). To correct for changes in electrode recording offsets between sessions, the mean voltage over the entire session was subtracted for every session and for every electrode after re-referencing.
Signal components
We analyzed the low and high frequency components of the recorded ECoG signals (Figure 4). The low frequency component was extracted by smoothing the preprocessed ECoG signals using a symmetric 2nd order Savitzky-Golay filter
The following popper user interface control may not be accessible. Tab to the next button to revert the control to an accessible version.
Destroy user interface control[33],The following popper user interface control may not be accessible. Tab to the next button to revert the control to an accessible version.
Destroy user interface control[34] with a time window of 250 ms (nominal 3 dB cut off frequency: 7.85 Hz for S1 and S2, 7.59 Hz for S3 and S4; estimated using table fromThe following popper user interface control may not be accessible. Tab to the next button to revert the control to an accessible version.
Destroy user interface control[35]; for justification on using this filter seeThe following popper user interface control may not be accessible. Tab to the next button to revert the control to an accessible version.
Destroy user interface control[16], section 1 of the supplementary material). We defined a window around each event of any kind, starting 3 seconds before the event and lasting until 3 seconds after it. The signals outside all of these windows were used as baseline activity. To enable a clear comparison to baseline, the average baseline activity was subtracted from the filtered recordings in each session for each electrode. The resulting signal was defined as the low frequency component of the signal (LFC).To analyze the high frequency component of the signal, time-resolved Fourier transformation using a Hamming window (333 ms window width, shifted in steps of 31 ms) was applied to the preprocessed signals, and the amplitudes were used for further analysis. To account for the general decrease in amplitude with increasing frequency, the amplitudes of every frequency bin were normalized by dividing them by the average baseline amplitude of the same frequency bin in the respective session. We then extracted the average amplitude across a frequency band from 60 Hz to 128 Hz for S1 and S2 and from 60 Hz to 200 Hz for S3 and S4. Since recordings for subjects S1 and S2 were sampled at 256 Hz, spectral amplitudes could be calculated only for frequencies up to 128 Hz (Nyquist frequency). Therefore, the frequency band used to calculate high frequency component could only comprise frequencies up to 128 Hz for subjects S1 and S2. Amplitudes calculated from ECoG signals recorded at least 3 seconds away from any event were used as baseline. To enable a clear comparison to baseline, the average baseline activity was subtracted from the extracted amplitudes in each session for each electrode. The resulting signal was defined as the high frequency component of the signal (HFC).
When LFC and HFC were used together for detection, we normalized every electrode and signal component to zero mean and unit variance, to accommodate for their different scaling.
Detection algorithm
To detect error events from the neural activity, we trained a set of classifiers that captured the neuronal features which are specific to error events (Figure 5). To be sure that the training data did not include neuronal responses to non-error events which were erroneously identified as ERNRs, we used ERNRs elicited by “clean” events only. Given a signal component Φ, the peri-error feature vector was defined as:
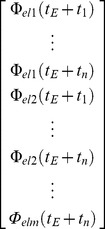
where tE is the time of an error event, el1, …, elm are the selected electrodes and t1, …, tn are the selected time points relative to the time of the error event. Therefore, the feature vector contains n•m
features for one signal component. If more than one signal component
was used, the feature vectors of the signal component were concatenated,
yielding an l•n•m dimensional feature vector, where l is the number of signal components used (l=
1 or 2 in this study). The time points t1, …, tn
were always equidistant and defined by a set of parameters: (i) the
time of the first feature in relation to the time of the error event, t1, (ii) the number of time points, n and (iii) the temporal distance between the first and the last feature, tn-t1.
Each classifier was build using two classes of feature vectors, the error class containing peri-error feature vectors (Eclass) and the baseline class containing feature vectors when no errors were present (Bclass).
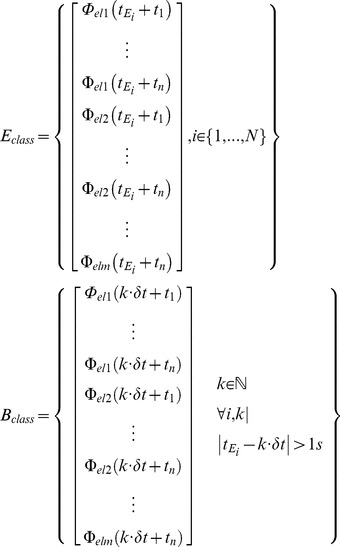
where δt is the time resolution of the signal component (LFC: 4 ms for S1 and S2, 1 ms for S3 and S4; 31 ms when HFC or both components were used). ”
These classes were used to build either a regularized linear discriminant analysis (rLDA) classifier or a regularized quadratic discriminant analysis (rQDA) classifier
The following popper user interface control may not be accessible. Tab to the next button to revert the control to an accessible version.
Destroy user interface control[36]. A QDA classifier is built by fitting a Gaussian distribution to each of the classes and gives the probability to belong to one of the classes for any arbitrary point in the feature space. Each Gaussian is represented by a class mean and a covariance matrix. LDA is a simplification of QDA, where fitted Gaussian distributions share a common covariance matrix. Regularization is implemented by modifying the covariance matrix of the fitted Gaussian distributions and has the purpose to improve the accuracy of the discriminant analyses on a new, independent set of data. We used the regularization of the form:
where C is the covariance matrix of the fitted Gaussian distribution, CR is the regularized covariance matrix, γ is the regularization coefficient and I is the identity matrix of the same size as C. In the case of rQDA, the number of vectors in the baseline class is much higher than the number of vectors in the error class. Therefore, we regularized the covariance matrix of the Gaussian fitted to the error class only. The classifier was then used to calculate the probability of every feature vector F in the test dataset to belong to the error class.

where tF is the time point corresponding to the calculated probability and tend is the duration of the tested dataset. We then extracted all local maxima of the probabilities and assigned a ‘detected as non-error’ (dne) state to remaining points. A threshold value λ was then selected and we assigned a dne state to all time points for which the value of the maxima remained below the threshold. Finally, we assigned a dne state to all remaining maxima for which there was a higher maximum less than 1 second away. We assigned a ‘detected as error’ (de) state to all remaining maxima. Since the classifier should be able to detect error events for which ERNRs were only slightly mixed with neuronal responses to other events, we calculated the detection measures between the times of detected events and the times of all real events within the test dataset, instead of just using the “clean” events.
To
properly validate the classifiers, we divided the recorded data into
three similarly long parts by splitting each session into three parts,
each containing one third of the “clean” events. First, we chose a set
of parameter values consisting of: (i) a time of the first feature in
relation to the error event, t1, (ii) a number of features, n and (iii) a time distance between the first and the last feature, tn-t1, (iv) regularization coefficient, γ, and (v) probability threshold λ.
An rLDA or rQDA classifier was then built using the first part of the
dataset. Using the built classifier, we detected the events on the
second part of the data and calculated the CYX.
Values of the parameters were then changed and the process was repeated,
until all parameter values from the parameter grid were tested. We used
the following grid of parameter values: (i) t1: from −667 ms to 667 ms in steps of 56 ms; (ii) n:
1, 3, 4, 5 and 8 when using single electrodes and electrode quartets
for detection and 1, 2 and 3 when using anatomical electrode subsets or
all grid electrodes for detection; (iii) tn-t1100 ms, 125 ms, 250 ms, 500 ms, 750 ms and 1000 ms; (iv) γ:
0, 0.01, 0.1, 0.3, 0.5, 0.7, 0.9, 0.99 and 1; and (v) probability
threshold: from 0.5 to 1 in steps of 0.017. The classifier that gave the
maximum CYX on the second part of the dataset was then used to detect the events on the third part of the dataset and TPR, FPR and CYX
were then calculated from this detection result. The same process was
repeated, now using the third part of the dataset for testing the grid
of parameter values and the second part of the dataset for classifier
testing. The average values of the two sets of TPR, FPR and CYX measures were then reported as the measured detection accuracy.
Different tolerance values were used to bin the experiment time into non-overlapping time bins, as described in section “Measures of detection accuracy”. The tolerance value directly determines the length of the dataset. Table 2 gives the dataset lengths for the tolerance values used.
MRNR subtraction
To remove the movement related neuronal responses (MRNRs) following a mismatch or a collision event, we used the MRNR subtraction method successfully applied in our previous study
The following popper user interface control may not be accessible. Tab to the next button to revert the control to an accessible version.
Destroy user interface control[16]. MRNR were identified by deriving and testing a set of classifiers relating the signals from one electrode to the movements using only non-event data (i.e. all data which was at least 1 s before and 3 s after any event). The most predictive classifier was selected and used to predict the MRNR for the whole recording, this time including the event data. MRNR signals were then subtracted from the initial signal, and the result was termed MRNR-free signals. All reported results were achieved by using MRNR-free signal for detection, unless specified otherwise.Neuroanatomical Analysis
To determine whether the motor or the somatosensory cortex played a more distinctive role in generating ERNR, we classified electrodes as motor cortex electrodes, somatosensory cortex electrodes, and other electrodes (Figure 3) in the same way as done in the previous study by Milekovic et al.
The following popper user interface control may not be accessible. Tab to the next button to revert the control to an accessible version.
Destroy user interface control[16].Statistical Analysis
All results are reported as mean ±95% confidence interval. To calculate confidence intervals, we used a bootstrap method with 10 000 re-samples
The following popper user interface control may not be accessible. Tab to the next button to revert the control to an accessible version.
Destroy user interface control[37]. Every reported statistics was bootstrapped separately for each of the subjects. For instance, if the difference between CYX was reported, we bootstrapped the difference directly. Subject-wise values of the statistics were considered as independent measurements and reported values were calculated using the error weighted meanThe following popper user interface control may not be accessible. Tab to the next button to revert the control to an accessible version.
Destroy user interface control[38].

where µi and σi are the subject-wise value of the statistics and its corresponding standard deviation estimated by bootstrapping, and µ and σ are the reported values of the statistics over subjects. We assumed that the measurements of the statistic were normally distributed and used the t-distribution to calculate the 95% confidence intervals
The following popper user interface control may not be accessible. Tab to the next button to revert the control to an accessible version.
Destroy user interface control[38].Results
Detection of Error Related Neuronal Responses
To
quantify how well outcome and execution events can be detected, we used
signals from all ECoG grid electrodes and in both signal components as
an input for our detection algorithm (Figure 6, ,7,7, see Figure 8
for topographical distribution of signals informative for error
detection). When detecting outcome error with a tolerance of 366 ms and
across all four subjects, average CYX was 0.69±0.04 with average TPR of 0.87±0.03 and average FPR of 0.24±0.04 (for individual subject values see Table 3). For detection of execution errors with the same tolerance, average CYX was 0.33±0.03 with average TPR of 0.64±0.04 and average FPR of 0.61±0.03 (for individual subject values see Table 3). Over all tolerance values, outcome error CYX values were higher than execution error CYX when both frequency components were used (CYX difference for tolerance of 366 ms: 0.36±0.05; for individual subjects: S10.58±0.08, S2
0.17±0.11, S3
0.15±0.12, S4
0.30±0.13).
Over all subjects, using both signal components gave significantly higher CYX then when using either one of the components alone for all tolerance values (CYX difference for tolerance of 366 ms over all subjects: outcome error: LFC & HFC vs. LFC: 0.23±0.05; LFC & HFC vs. HFC: 0.16±0.05; execution error: LFC & HFC vs. LFC: 0.15±0.04; LFC & HFC vs. HFC: 0.05±0.04). Using HCF gave significantly higher CYX than using LFC for tolerances of 366 ms and higher (CYX difference for tolerance of 366 ms: outcome error: 0.07±0.05; execution error: 0.09±0.03).
Topographical Distribution of Informative Signals for Error Detection
To determine the topographical distribution of signals that were informative for the error detection, we performed detection using signals recorded from electrode quartets (Figure 5). For most of the subjects, several isolated, often spatially quite distant peaks of CYX could be found over the cortical regions we recorded from. Locations of these peaks often differed for different error types and different signal components. Thus, we did not notice any topographical location that was systematically beneficial for detecting either outcome or execution errors.
Error Detection Using Signals from Motor or Somatosensory Areas
We compared the performance of error detection based on recordings from different anatomical subareas (motor, somatosensory and other areas) to the error detection performance based on recordings from all channels (Figure 9). For all 4 participants, the detection performance was highest when all electrodes were used; with subarea electrode sets reaching in some cases an equivalent performance.
Next, we tested if recordings from motor or somatosensory areas provided enough information for high accuracy of error detection. To this end, we calculated the percentage of CYX achieved when using signals from these areas compared to the CYX values achieved when using all ECoG grid electrodes. These percentages were first averaged over all tolerance values and then over subjects. For S2, motor and somatosensory cortex was not well covered with electrodes as only 3 or 2 electrodes recorded from these areas (Table 4). We, therefore, excluded S2 from this analysis. Detection performance from motor cortex signals was 75±2% and 77±4% of the total detection performance for outcome and execution error respectively. Performance from somatosensory cortex signals reached 63±2% (outcome error) and 50±3% (execution error) of the total performance.
Detection from Smaller Electrode Sets
We investigated whether one can detect error events with smaller electrode subsets with accuracy similar to detection when all ECoG grid electrodes were used (Figure 10). When both frequency components were used for the tolerance of 366 ms, maximum CYX from single electrodes was 60±6% for outcome error and 66±9% for execution error of the CYX when all electrodes were used. For electrode quartets and both frequency components, maximum CYX was 87±6% for outcome error and 78±10% for execution error of CYX when all electrodes were used.
Effect of MRNR Subtraction on the Normalized Mutual Information
We also investigated whether MRNR subtraction affected the detection of the error events (Figure 11). Over all subjects and tolerance values, the difference in CYX when MRNR subtraction was and was not used was not significant when both LFC and HFC were used for detection, except for tolerances of 472 ms and 1 s for execution error for which the signals without MRNR subtraction gave higher CYX values (CYX difference for tolerance of 366 ms: outcome: −0.01±0.05; execution: 0.00±0.04). When only LFC was used for detection, using MRNR subtraction lead to a slight, but significant improvement, except for execution error for tolerances of 155 ms and 261 ms (CYX difference for tolerance of 366 ms: outcome error: −0.14±0.04; execution error: −0.07±0.03). For the high frequency component, using MRNR subtraction did not change the CYX values, except for outcome error for tolerances of 155 ms and 894 ms, for which the CYX values were slightly significantly worse (CYX difference for tolerance of 366 ms: outcome error: 0.03±0.05; execution error: 0.00±0.04).
Selection of the Classifier Type for Detection: rLDA vs. rQDA
We compared the detection performance between rLDA and rQDA (Figure 12). rQDA is more flexible, but has the drawback that more free parameters need to be estimated from the training dataset. The number of free parameters is a quadratic function of the number of signal features, which, in turn, is the product of the number of electrodes and the number of features taken from each single electrode. Therefore, if the dataset is quite large and the total number of features used to build the classifier is quite small, rQDA might outperform rLDA. On the other hand, if the data is limited and the total number of features is high, rLDA might outperform rQDA. We wanted to determine in which one of these two regimes our dataset was.
If signals from single electrodes were used for detection, rLDA and rQDA yielded essentially the same performance, with differences being small compared to the performance of any of the classifiers and, in many cases, insignificant (Figure 12). If signals from electrode quartets were used, rLDA performance was in most cases significantly higher than rQDA, but always at least as high as rQDA. For all electrodes, rLDA always significantly outperformed rQDA.
Discussion
In our previous study
The following popper user interface control may not be accessible. Tab to the next button to revert the control to an accessible version.
Destroy user interface control[16] we demonstrated that the ECoG signals used here for error detection indeed reflect outcome and execution ERNRs and are not related to movements or caused by visual stimuli or surprise effects. Here, we showed that both outcome and execution error events can be reliably detected from continuous neuronal activity measured with ECoG electrode implants. These results suggest that, for both error types, it is possible to detect more than half of the errors and that the number of false positive detections is comparable to the number of true positive detections. In our experiment, error events were extremely rare. Therefore, these results suggest that error events can be detected with high reliability. This notion is supported by the relatively high values of the normalized mutual information.Signals informative about the times of error events were not found in one isolated cortical area only, but rather in multiple different areas (Figure 8). Often, it was possible to find several, mutually isolated CYX peaks in one subject for the same error type and the same frequency component. In addition, CYX peaks often had different locations for different error types and different location for different frequency components. This suggests that neuronal responses coding for outcome errors might be independent from the neuronal responses coding for execution errors. In addition, it suggests that low and high frequency component of the neuronal responses coding for the same error might also be independent. This is consistent with results in the previous study by Milekovic et al.
The following popper user interface control may not be accessible. Tab to the next button to revert the control to an accessible version.
Destroy user interface control[16], where it was shown that the topographical distribution of ERNR’s signal to noise ratio had similar, distributed properties.We also tested whether signals from a particular anatomical area gave more or less informative signals for detection. To this end, we divided the ECoG electrodes for each of the subjects into three subsets: motor set, somatosensory set and the ‘other’ set. In S2, the ECoG grid was implanted more ventrally than in other subjects, thereby covering only the ventral motor and somatosensory areas. In addition, ESM was not performed on S2. Therefore, for S2, we had to use anatomical reconstruction of major cortical sulci locations from the patients’ MRI scans to determine which electrodes belonged to motor and somatosensory subsets. This resulted in a motor set of only 3 electrodes and a somatosensory set of only 2 electrodes (Table 4). Due to the low number of electrodes in these subsets, and due to the more ventral location of the ECoG implant, we decided that detection results of motor and somatosensory sets for S2 were not representative as these areas were not sufficiently covered and, therefore, excluded S2 from the anatomical area specific analysis. For the remaining three subjects, none of the anatomical subsets provide better signals for detecting outcome errors. In the case of execution error, detection using motor and other electrode subsets was similar and only a bit better then detection from the somatosensory subset.
A complete 8×8 electrode ECoG grid covers a surface of around 64 cm2. To implant such grids, a craniotomy of similar size is required. Studies on risk factors of subchronic implantations for pre-neurosurgical epilepsy diagnostics indicate that the size of the implant is a risk factor for complications of subdural ECoG implantations
The following popper user interface control may not be accessible. Tab to the next button to revert the control to an accessible version.
Destroy user interface control[39]. Therefore, we investigated whether similar detection accuracy could be obtained using signals from smaller parts of the grid only. Signals from single electrodes gave much lower detection accuracy in comparison to using all ECoG electrodes but, nevertheless, more than 60% of the CYX value when using all grid electrodes could be obtained for any of the error types. Detection based on signals from electrode quartets (2×2 neighbouring electrodes) almost reached the level of detection obtained by using signals from all electrodes, obtaining more than 78% of the CYX value when using all grid electrodes for any of the error types. These results suggest that, if the optimal location for an implant is known in advance, one could safely reduce the size of the electrode by 16 fold, with only a small loss in detection accuracy.Besides reducing medical risks, there are additional benefits when reducing the number of electrodes used for error detection. The numerical complexity of the detection algorithm is a quadratic function of the number of used electrodes. Therefore, reducing the number of electrodes by 16 fold could reduce the numerical complexity of the detection algorithm by up to 256 times, which would drastically reduce the computational requirements of the detection process, allowing the process to be run on less powerful computers, or freeing computational resources for other tasks. To determine which electrodes or electrode quartets would be best for detection, one could run the entire detection process on a set of data recorded for such calibration purposes. On the other hand, such an optimization process might take a considerable amount of computation time.
A high proportion of our electrodes were located above the motor and somatosensory cortical areas. Therefore, it should be expected that some fraction of our signals contained movement related neuronal signals. In Milekovic et al.
The following popper user interface control may not be accessible. Tab to the next button to revert the control to an accessible version.
Destroy user interface control[16], analysis revealed that large amounts of the variance on some of the electrodes could be explained by a classifier predicting neuronal signals from eye and hand movements. This study than removed this proportion of the signals by subtracting the movement related signals predicted by such classifiers. Here, we performed error detection using the same MRNR subtraction procedure and compared it to the detection accuracy when error detection was performed without MRNR subtraction. We found that, on average, MRNR subtraction did not significantly change the detection accuracy, demonstrating that our error detection was really based on error related and not on any movement related neuronal signals.We also compared detection accuracy between detection using rLDA and rQDA. In our experiment, subjects performed during about one hour of recordings, which left about 20 minutes of recordings for training the detector. In these experimental conditions, the detector using rLDA clearly outperformed the detector using rQDA when signals from all ECoG electrodes were used. In this case, the number of possible features used for detection greatly outnumbered the number of ERNR examples used for building the classifier. Since rQDA classifiers need to estimate a higher number of parameters than rLDA classifiers, it is no surprise that detection based on rLDA showed better results. On the other hand, when signals from only a single electrodes and a single signal component were used for detection, using rQDA was as accurate or better than using rLDA. In this case of low numbers of features, the detection benefited from the higher flexibility of rQDA classifiers. But, when using signals from only 4 electrodes, detection accuracy was significantly higher when using rLDA. For larger datasets containing more ERNR examples that could be used for detection classifier building, rQDA might further outperform rLDA classifiers although, based on our studies, one would rather include larger number of channels since, by doing so, the gain of detection accuracy becomes higher.
Characteristics of Signal Components Used for Detection
Here, we used both low (0–8 Hz) and high (60–128 Hz for S1 and S2 and 60–200 Hz for S3 and S4) frequency components of the neuronal signals to detect the error events. According to the study of Milekovic et al.
The following popper user interface control may not be accessible. Tab to the next button to revert the control to an accessible version.
Destroy user interface control[16], these signal components gave two different, possibly independent, sources of information about errors. Other studies showed that neuronal responses with similar spectro-temporal characteristics can be evoked by non-error events, such as different movementsThe following popper user interface control may not be accessible. Tab to the next button to revert the control to an accessible version.
Destroy user interface control[40]–The following popper user interface control may not be accessible. Tab to the next button to revert the control to an accessible version.
Destroy user interface control[46], somatosensory and auditory stimuliThe following popper user interface control may not be accessible. Tab to the next button to revert the control to an accessible version.
Destroy user interface control[47]–The following popper user interface control may not be accessible. Tab to the next button to revert the control to an accessible version.
Destroy user interface control[49], word recognitionThe following popper user interface control may not be accessible. Tab to the next button to revert the control to an accessible version.
Destroy user interface control[50], face recognitionThe following popper user interface control may not be accessible. Tab to the next button to revert the control to an accessible version.
Destroy user interface control[51] and attention and short term memoryThe following popper user interface control may not be accessible. Tab to the next button to revert the control to an accessible version.
Destroy user interface control[52]. One could argue that detecting ERNR in a more natural environment compared to our, highly controlled task will be more challenging as the ERNRs might not be differentiable from neural responses to non-error events. This should, however, not necessarily hinder the applicability of our error detection appoach. If subjects are focused on the task at hand, they are expected to perform only minimal amounts of additional movements, receive minimal amounts of additional tactile stimuli and will probably not perform additional cognitive tasks. In our task, subjects were merely asked not to move too much and to try to remain focused on the task. We argue that they would show similar behavior if they were motivated to perform the task for their personal benefit, such as navigating the cursor or artificial hand towards the target. Therefore, we think that the ECoG signal can also be applied for online continuous error detection under more natural conditions.Detection of error events could still be possible, even in environments with more somatosensory stimuli and more different tasks at once. ERNRs used in this study were evoked on multiple, often quite distant, electrodes and these evoked responses exhibited quite different time courses. This makes the ERNR responses highly redundant and likely differentiable to other, non-error neuronal responses. Some of the earlier mentioned studies already used these signal properties to differentiate between neuronal responses to different non-error events
The following popper user interface control may not be accessible. Tab to the next button to revert the control to an accessible version.
Destroy user interface control[40],The following popper user interface control may not be accessible. Tab to the next button to revert the control to an accessible version.
Destroy user interface control[41],The following popper user interface control may not be accessible. Tab to the next button to revert the control to an accessible version.
Destroy user interface control[49] and the same principle could work for ERNRs as well. Further studies are required to test the accuracy of error detection in such more complex environments.Comparison to Previous Detection Studies
Several studies investigated the detection of epileptic seizures from neuronal recordings
The following popper user interface control may not be accessible. Tab to the next button to revert the control to an accessible version.
Destroy user interface control[53],The following popper user interface control may not be accessible. Tab to the next button to revert the control to an accessible version.
Destroy user interface control[54]. Since epileptic seizures occur very rarely and cause hospital staff alarms during the epileptic assessment periods, there is a strong requirement to keep the number of false positive detections at a minimum. Therefore, when measuring the accuracy of seizure detections, TPR is usually combined with the number of false positives per hourThe following popper user interface control may not be accessible. Tab to the next button to revert the control to an accessible version.
Destroy user interface control[55]. Since the frequency of decoding errors during continuous control with current BMI approaches is much higher than the typical number of epileptic seizures, using the number of false positives per hour as a measure of performance does not apply well to error detection. A number of other studies used neuronal signals to detect movement related events, such as movement onsetThe following popper user interface control may not be accessible. Tab to the next button to revert the control to an accessible version.
Destroy user interface control[56]–The following popper user interface control may not be accessible. Tab to the next button to revert the control to an accessible version.
Destroy user interface control[59], movement planning phaseThe following popper user interface control may not be accessible. Tab to the next button to revert the control to an accessible version.
Destroy user interface control[60] and periods of movement related synchronization and desynchronizationThe following popper user interface control may not be accessible. Tab to the next button to revert the control to an accessible version.
Destroy user interface control[27]. These studies mainly used TPR and FPR to visualize the detection results, while some of them used the Youden index i

The following popper user interface control may not be accessible. Tab to the next button to revert the control to an accessible version.
Destroy user interface control[61] as a single measure of detection performanceThe following popper user interface control may not be accessible. Tab to the next button to revert the control to an accessible version.
Destroy user interface control[56],The following popper user interface control may not be accessible. Tab to the next button to revert the control to an accessible version.
Destroy user interface control[59]. Solis-Escalante et al.The following popper user interface control may not be accessible. Tab to the next button to revert the control to an accessible version.
Destroy user interface control[27] used mutual information to report their final results, but still used the Youden index to calibrate their detector. Here, due to its strong theoretical foundations, we used normalized mutual information to both calibrate the detector and measure its accuracy.The afore mentioned studies used a wide variety of algorithms for detection: expectation maximization Gaussian mixture classifier
The following popper user interface control may not be accessible. Tab to the next button to revert the control to an accessible version.
Destroy user interface control[59], k nearest neighborsThe following popper user interface control may not be accessible. Tab to the next button to revert the control to an accessible version.
Destroy user interface control[57], linear discriminant analysisThe following popper user interface control may not be accessible. Tab to the next button to revert the control to an accessible version.
Destroy user interface control[57], local field potential β-band power threshold crossingThe following popper user interface control may not be accessible. Tab to the next button to revert the control to an accessible version.
Destroy user interface control[58], cross-correlation threshold crossingThe following popper user interface control may not be accessible. Tab to the next button to revert the control to an accessible version.
Destroy user interface control[56], support vector machinesThe following popper user interface control may not be accessible. Tab to the next button to revert the control to an accessible version.
Destroy user interface control[27], recursive Bayesian classifierThe following popper user interface control may not be accessible. Tab to the next button to revert the control to an accessible version.
Destroy user interface control[60] and phase slope index threshold crossingThe following popper user interface control may not be accessible. Tab to the next button to revert the control to an accessible version.
Destroy user interface control[54]. Here, we used regularized versions of linear and quadratic discriminant analyses with a variable threshold for detection. This makes our algorithms linear or quadratic in nature. Use of more complex and flexible algorithms might improve the detection, although, in the present study, the simpler rLDA algorithm outperformed the more complex rQDA algorithm whenever more than one electrode or signal component was used.The afore mentioned studies detecting movement states did not investigate the effect of temporal tolerance on the precision of the detector. Here, we showed that detection accuracy rises with the tolerance, until it saturates at around 300–500 ms. This implies that ERNRs used to detect error events are not perfectly timed to those events, and that the variability or ERNRs in response to error events is at the level of 300–500 ms. Reasons for this variability might be imprecision due to noise or other kinds of neuronal activity (e.g. ongoing activity) or the variability in the time subjects needed to recognize the error. Measurements of choice reaction time variability
The following popper user interface control may not be accessible. Tab to the next button to revert the control to an accessible version.
Destroy user interface control[62] have reported reaction time variability of about 400 ms (95% of all reactions). In any case, the variability caused by the limited detection frequency of 32 Hz should be negligible compared to other effects.Comparison to Previous ERNR Studies
Most of the earlier ERNR studies concentrated on activity of the anterior cingulate cortex (ACC) and its functional significance
The following popper user interface control may not be accessible. Tab to the next button to revert the control to an accessible version.
Destroy user interface control[63]–The following popper user interface control may not be accessible. Tab to the next button to revert the control to an accessible version.
Destroy user interface control[65]. These studies were conducted using trial-based tasks measuring brain activity by the electroencephalogram (EEG). The observed neuronal responses were classified into several types: response error related negativity (rERN)The following popper user interface control may not be accessible. Tab to the next button to revert the control to an accessible version.
Destroy user interface control[66],The following popper user interface control may not be accessible. Tab to the next button to revert the control to an accessible version.
Destroy user interface control[67], feedback error related negativity (fERN)The following popper user interface control may not be accessible. Tab to the next button to revert the control to an accessible version.
Destroy user interface control[68], observation error potential (oErrP)The following popper user interface control may not be accessible. Tab to the next button to revert the control to an accessible version.
Destroy user interface control[69] and interaction error potential (iErrP)The following popper user interface control may not be accessible. Tab to the next button to revert the control to an accessible version.
Destroy user interface control[70]. In contrast to these studies, we investigated errors during a continuous task. At this stage, it is unclear what the relation between the mechanisms generating these two kinds of responses is or whether the mechanisms are different at all. Krigolson and HolroydThe following popper user interface control may not be accessible. Tab to the next button to revert the control to an accessible version.
Destroy user interface control[15],The following popper user interface control may not be accessible. Tab to the next button to revert the control to an accessible version.
Destroy user interface control[18] compared EEG correlates of error-related activity during a continuous tracking task to the ERNs observed in trial-based tasks of previous studiesThe following popper user interface control may not be accessible. Tab to the next button to revert the control to an accessible version.
Destroy user interface control[67],The following popper user interface control may not be accessible. Tab to the next button to revert the control to an accessible version.
Destroy user interface control[68]. Krigolson and Holroyd report similarities (e.g. spatial distribution of the neuronal error signal) and differences (e.g. in the timing of the response) between the continuous error responses recorded in their study and trial-based rERN and fERN reported in other studies. However, in contrast to our study they use EEG instead of ECoG and their continuous task is also different from ours. Thus, further investigations would be needed to clarify the relation between the ERNRs reported in our study and the well-established ERN. To address this interesting question, one could compare trial-based and continuous error responses in the same subjects using the same recording methodology. To the best of our knowledge, no such study has been carried out so far and we consider this to be an interesting topic for future research.ERNRs have also previously been found in motor cortex
The following popper user interface control may not be accessible. Tab to the next button to revert the control to an accessible version.
Destroy user interface control[14],The following popper user interface control may not be accessible. Tab to the next button to revert the control to an accessible version.
Destroy user interface control[16],The following popper user interface control may not be accessible. Tab to the next button to revert the control to an accessible version.
Destroy user interface control[69],The following popper user interface control may not be accessible. Tab to the next button to revert the control to an accessible version.
Destroy user interface control[71], somatosensory cortexThe following popper user interface control may not be accessible. Tab to the next button to revert the control to an accessible version.
Destroy user interface control[14],The following popper user interface control may not be accessible. Tab to the next button to revert the control to an accessible version.
Destroy user interface control[16] and in other cortical areasThe following popper user interface control may not be accessible. Tab to the next button to revert the control to an accessible version.
Destroy user interface control[14],The following popper user interface control may not be accessible. Tab to the next button to revert the control to an accessible version.
Destroy user interface control[16],The following popper user interface control may not be accessible. Tab to the next button to revert the control to an accessible version.
Destroy user interface control[72]–The following popper user interface control may not be accessible. Tab to the next button to revert the control to an accessible version.
Destroy user interface control[77]. Our study extends our previous findingsThe following popper user interface control may not be accessible. Tab to the next button to revert the control to an accessible version.
Destroy user interface control[16] by showing that error related neuronal responses recorded by ECoG can be detected in continuous neuronal activity recordings. Furthermore, we demonstrated that outcome errors could be detected with higher accuracy than execution errors.Relevance for Brain Machine Interfaces
One motivation for this study was to investigate whether ERNRs can be used to improve the performance of continuous movement BMIs
The following popper user interface control may not be accessible. Tab to the next button to revert the control to an accessible version.
Destroy user interface control[1],The following popper user interface control may not be accessible. Tab to the next button to revert the control to an accessible version.
Destroy user interface control[3],The following popper user interface control may not be accessible. Tab to the next button to revert the control to an accessible version.
Destroy user interface control[8],The following popper user interface control may not be accessible. Tab to the next button to revert the control to an accessible version.
Destroy user interface control[9],The following popper user interface control may not be accessible. Tab to the next button to revert the control to an accessible version.
Destroy user interface control[78]–The following popper user interface control may not be accessible. Tab to the next button to revert the control to an accessible version.
Destroy user interface control[80].To achieve this goal, (i) error related neuronal signals need to be present in the used recording of neuronal activity, (ii) these error related signals need to be detectable, (iii) different error types (e.g. execution and outcome error) need to be differentiable from the signals and (iv) adaptive decoding algorithms utilizing error signals need to be available. Extensive research in error related neuronal signals already showed that such signals can be recorded with a wide range of recording techniques. Specifically, Milekovic et al.
The following popper user interface control may not be accessible. Tab to the next button to revert the control to an accessible version.
Destroy user interface control[16] showed that error signals can be recorded using ECoG during continuous tasks and that execution errors can be differentiated from outcome errors, thereby resolving points (i) and (iii) for ECoG based BMIs. Adaptive algorithms decoding continuous movements can indeed benefit from error detectionThe following popper user interface control may not be accessible. Tab to the next button to revert the control to an accessible version.
Destroy user interface control[12],The following popper user interface control may not be accessible. Tab to the next button to revert the control to an accessible version.
Destroy user interface control[81], resolving point (iv). In this study, we showed that error detection is possible, thereby resolving point (ii). Even though all points have now been resolved, it still remains necessary to demonstrate the proposed continuous BMI decoding system that utilizes neuronal error signals in an online study, making this an interesting topic for future research.Our previous study
The following popper user interface control may not be accessible. Tab to the next button to revert the control to an accessible version.
Destroy user interface control[16] showed that outcome and execution errors can be reliably distinguished upon detection. Instead of just providing binary information, whether a decoding error has been made or not, detection of multiple error types could further improve BMI operation. Outcome error detection can be used to subsequently correct the errorsThe following popper user interface control may not be accessible. Tab to the next button to revert the control to an accessible version.
Destroy user interface control[5]–The following popper user interface control may not be accessible. Tab to the next button to revert the control to an accessible version.
Destroy user interface control[7],The following popper user interface control may not be accessible. Tab to the next button to revert the control to an accessible version.
Destroy user interface control[70],The following popper user interface control may not be accessible. Tab to the next button to revert the control to an accessible version.
Destroy user interface control[82]. Subsequently correcting decoded trajectory errors by detecting execution errors might not be beneficial, since BMI users will rather try to correct the decoding mistake by corrective movements. On the other hand, in a task where movements are made to perform some kind of selection, e.g. by guiding a cursor to one of several target locations, it would be possible to subsequently correct incorrect selections by detecting outcome errors, thereby making the BMI more efficient. In addition, outcome errors can also be used within the reinforcement learning frameworkThe following popper user interface control may not be accessible. Tab to the next button to revert the control to an accessible version.
Destroy user interface control[83]. However, reinforcement learning algorithms tend to require long recording sessions, making them much slower in their adaptation towards more efficient BMI decoders.Detection of execution errors can be used to directly label incorrectly decoded trajectories and, thus, the part of the recordings that were incorrectly interpreted. Such information can be used to facilitate BMI algorithm adaptation
The following popper user interface control may not be accessible. Tab to the next button to revert the control to an accessible version.
Destroy user interface control[12],The following popper user interface control may not be accessible. Tab to the next button to revert the control to an accessible version.
Destroy user interface control[81].Even though we carried out offline analyses in this study, our detection methods are directly applicable to detect errors in online experiments and could be utilized as a part of an online BMI system. For intermediate channel and feature numbers no special computer hardware will be required; in fact similar preprocessing and decoding algorithms
The following popper user interface control may not be accessible. Tab to the next button to revert the control to an accessible version.
Destroy user interface control[23],The following popper user interface control may not be accessible. Tab to the next button to revert the control to an accessible version.
Destroy user interface control[84]–The following popper user interface control may not be accessible. Tab to the next button to revert the control to an accessible version.
Destroy user interface control[86] as utilized by our detection algorithm, have already been used in real-time during online BMI applications on standard computing hardware. For high channel/feature numbers parallel processing, e.g. utilizing the graphics processing unit or multiple cores of standard laptop/desktop computers, might be usedThe following popper user interface control may not be accessible. Tab to the next button to revert the control to an accessible version.
Destroy user interface control[87].For most invasive BMIs, motor cortex is the primary target area for the implantation of electrodes whose signals are to be used to extract intended motor actions. We demonstrated that one can detect error signals with high accuracy based on motor cortical signals only. Therefore, movement decoding and error detection may be implemented using the same electrode implants. Consequently, no additional implants over other cortical areas would be required for BMIs employing such neuronal error signals, thereby not inflicting additional medical risk when adding error detection to a BMI system.
Acknowledgments
We would like to thank the subjects for participating in our study. We are grateful to the staff of the Freiburg University Hospital, Epilepsy Center for their help.
Funding Statement
References
Formats:
- Article |
- PubReader |
- ePub (beta) |
- PDF (3.1M)
- Error-related electrocorticographic activity in humans during continuous movements.[J Neural Eng. 2012]Milekovic TBall T, Schulze-Bonhage A, Aertsen A, Mehring C, . J Neural Eng. 2012 Apr; 9(2):026007. Epub 2012 Feb 13.
- Recording human electrocorticographic (ECoG) signals for neuroscientific research and real-time functional cortical mapping.[J Vis Exp. 2012]Hill NJGupta D, Brunner P, Gunduz A, Adamo MA, Ritaccio A, Schalk G, . J Vis Exp. 2012 Jun 26; (64). Epub 2012 Jun 26.
- Real-time control of a prosthetic hand using human electrocorticography signals.[J Neurosurg. 2011]Yanagisawa THirata M, Saitoh Y, Goto T, Kishima H, Fukuma R, Yokoi H, Kamitani Y, Yoshimine T, . J Neurosurg. 2011 Jun; 114(6):1715-22. Epub 2011 Feb 11.
- A review on directional information in neural signals for brain-machine interfaces.[J Physiol Paris. 2009]Waldert SPistohl T, Braun C, Ball T, Aertsen A, Mehring C, . J Physiol Paris. 2009 Sep-Dec; 103(3-5):244-54. Epub 2009 Aug 7.
- High-frequency gamma oscillations and human brain mapping with electrocorticography.[Prog Brain Res. 2006]Crone NESinai A, Korzeniewska A, . Prog Brain Res. 2006; 159:275-95.
- PubMedPubMedPubMed citations for these articles
- Detection of Error Related Neuronal Responses Recorded by Electrocorticography i...Detection of Error Related Neuronal Responses Recorded by Electrocorticography in Humans during Continuous MovementsPLoS ONE. 2013; 8(2)PMC
Your browsing activity is empty.
Activity recording is turned off.
See more...- Cortical control of a prosthetic arm for self-feeding.[Nature. 2008]Velliste M, Perel S, Spalding MC, Whitford AS, Schwartz ABNature. 2008 Jun 19; 453(7198):1098-101.
- Neural control of computer cursor velocity by decoding motor cortical spiking activity in humans with tetraplegia.[J Neural Eng. 2008]Kim SP, Simeral JD, Hochberg LR, Donoghue JP, Black MJJ Neural Eng. 2008 Dec; 5(4):455-76.
- EEG-based communication: presence of an error potential.[Clin Neurophysiol. 2000]Schalk G, Wolpaw JR, McFarland DJ, Pfurtscheller GClin Neurophysiol. 2000 Dec; 111(12):2138-44.
- Online detection of error-related potentials boosts the performance of mental typewriters.[BMC Neurosci. 2012]Schmidt NM, Blankertz B, Treder MSBMC Neurosci. 2012 Feb 15; 13():19.
- Learning to control a brain-machine interface for reaching and grasping by primates.[PLoS Biol. 2003]Carmena JM, Lebedev MA, Crist RE, O'Doherty JE, Santucci DM, Dimitrov DF, Patil PG, Henriquez CS, Nicolelis MAPLoS Biol. 2003 Nov; 1(2):E42.
- Reach and grasp by people with tetraplegia using a neurally controlled robotic arm.[Nature. 2012]Hochberg LR, Bacher D, Jarosiewicz B, Masse NY, Simeral JD, Vogel J, Haddadin S, Liu J, Cash SS, van der Smagt P, Donoghue JPNature. 2012 May 16; 485(7398):372-5.
- Neuronal ensemble control of prosthetic devices by a human with tetraplegia.[Nature. 2006]Hochberg LR, Serruya MD, Friehs GM, Mukand JA, Saleh M, Caplan AH, Branner A, Chen D, Penn RD, Donoghue JPNature. 2006 Jul 13; 442(7099):164-71.
- Neural
control of cursor trajectory and click by a human with tetraplegia 1000
days after implant of an intracortical microelectrode array.[J Neural Eng. 2011]Simeral JD, Kim SP, Black MJ, Donoghue JP, Hochberg LRJ Neural Eng. 2011 Apr; 8(2):025027.
- Towards on-line adaptation of neuro-prostheses with neuronal evaluation signals.[Biol Cybern. 2006]Rotermund D, Ernst UA, Pawelzik KRBiol Cybern. 2006 Sep; 95(3):243-57.
- Adaptive classification for brain computer interfaces.[Conf Proc IEEE Eng Med Biol Soc. 2007]Blumberg J, Rickert J, Waldert S, Schulze-Bonhage A, Aertsen A, Mehring CConf Proc IEEE Eng Med Biol Soc. 2007; 2007():2536-9.
- Neural correlates of reach errors.[J Neurosci. 2005]Diedrichsen J, Hashambhoy Y, Rane T, Shadmehr RJ Neurosci. 2005 Oct 26; 25(43):9919-31.
- Hierarchical error processing: different errors, different systems.[Brain Res. 2007]Krigolson OE, Holroyd CBBrain Res. 2007 Jun 25; 1155():70-80.
- Error-related electrocorticographic activity in humans during continuous movements.[J Neural Eng. 2012]Milekovic T, Ball T, Schulze-Bonhage A, Aertsen A, Mehring CJ Neural Eng. 2012 Apr; 9(2):026007.
- Predictive information and error processing: the role of medial-frontal cortex during motor control.[Psychophysiology. 2007]Krigolson OE, Holroyd CBPsychophysiology. 2007 Jul; 44(4):586-95.
- Review Evolution of brain-computer interface: action potentials, local field potentials and electrocorticograms.[Curr Opin Neurobiol. 2010]Moran DCurr Opin Neurobiol. 2010 Dec; 20(6):741-5.
- Two-dimensional movement control using electrocorticographic signals in humans.[J Neural Eng. 2008]Schalk G, Miller KJ, Anderson NR, Wilson JA, Smyth MD, Ojemann JG, Moran DW, Wolpaw JR, Leuthardt ECJ Neural Eng. 2008 Mar; 5(1):75-84.
- Decoding flexion of individual fingers using electrocorticographic signals in humans.[J Neural Eng. 2009]Kubánek J, Miller KJ, Ojemann JG, Wolpaw JR, Schalk GJ Neural Eng. 2009 Dec; 6(6):066001.
- Decoding natural grasp types from human ECoG.[Neuroimage. 2012]Pistohl T, Schulze-Bonhage A, Aertsen A, Mehring C, Ball TNeuroimage. 2012 Jan 2; 59(1):248-60.
- Error-related electrocorticographic activity in humans during continuous movements.[J Neural Eng. 2012]Milekovic T, Ball T, Schulze-Bonhage A, Aertsen A, Mehring CJ Neural Eng. 2012 Apr; 9(2):026007.
- Error-related electrocorticographic activity in humans during continuous movements.[J Neural Eng. 2012]Milekovic T, Ball T, Schulze-Bonhage A, Aertsen A, Mehring CJ Neural Eng. 2012 Apr; 9(2):026007.
- Error-related electrocorticographic activity in humans during continuous movements.[J Neural Eng. 2012]Milekovic T, Ball T, Schulze-Bonhage A, Aertsen A, Mehring CJ Neural Eng. 2012 Apr; 9(2):026007.
- Error-related electrocorticographic activity in humans during continuous movements.[J Neural Eng. 2012]Milekovic T, Ball T, Schulze-Bonhage A, Aertsen A, Mehring CJ Neural Eng. 2012 Apr; 9(2):026007.
- Rapid and fully automated visualization of subdural electrodes in the presurgical evaluation of epilepsy patients.[AJNR Am J Neuroradiol. 2005]Kovalev D, Spreer J, Honegger J, Zentner J, Schulze-Bonhage A, Huppertz HJAJNR Am J Neuroradiol. 2005 May; 26(5):1078-83.
- Overt foot movement detection in one single Laplacian EEG derivation.[J Neurosci Methods. 2008]Solis-Escalante T, Müller-Putz G, Pfurtscheller GJ Neurosci Methods. 2008 Oct 30; 175(1):148-53.
- Overt foot movement detection in one single Laplacian EEG derivation.[J Neurosci Methods. 2008]Solis-Escalante T, Müller-Putz G, Pfurtscheller GJ Neurosci Methods. 2008 Oct 30; 175(1):148-53.
- Asymptotic bias in information estimates and the exponential (Bell) polynomials.[Neural Comput. 2000]Victor JDNeural Comput. 2000 Dec; 12(12):2797-804.
- Smoothing and differentiation of data by simplified least square procedure.[Anal Chem. 1972]Steinier J, Termonia Y, Deltour JAnal Chem. 1972 Sep 1; 44(11):1906-9.
- Error-related electrocorticographic activity in humans during continuous movements.[J Neural Eng. 2012]Milekovic T, Ball T, Schulze-Bonhage A, Aertsen A, Mehring CJ Neural Eng. 2012 Apr; 9(2):026007.
- Error-related electrocorticographic activity in humans during continuous movements.[J Neural Eng. 2012]Milekovic T, Ball T, Schulze-Bonhage A, Aertsen A, Mehring CJ Neural Eng. 2012 Apr; 9(2):026007.
- Error-related electrocorticographic activity in humans during continuous movements.[J Neural Eng. 2012]Milekovic T, Ball T, Schulze-Bonhage A, Aertsen A, Mehring CJ Neural Eng. 2012 Apr; 9(2):026007.
- Error-related electrocorticographic activity in humans during continuous movements.[J Neural Eng. 2012]Milekovic T, Ball T, Schulze-Bonhage A, Aertsen A, Mehring CJ Neural Eng. 2012 Apr; 9(2):026007.
- Error-related electrocorticographic activity in humans during continuous movements.[J Neural Eng. 2012]Milekovic T, Ball T, Schulze-Bonhage A, Aertsen A, Mehring CJ Neural Eng. 2012 Apr; 9(2):026007.
- Risk
factors for complications during intracranial electrode recording in
presurgical evaluation of drug resistant partial epilepsy.[Acta Neurochir (Wien). 2009]Wong CH, Birkett J, Byth K, Dexter M, Somerville E, Gill D, Chaseling R, Fearnside M, Bleasel AActa Neurochir (Wien). 2009 Jan; 151(1):37-50.
- Error-related electrocorticographic activity in humans during continuous movements.[J Neural Eng. 2012]Milekovic T, Ball T, Schulze-Bonhage A, Aertsen A, Mehring CJ Neural Eng. 2012 Apr; 9(2):026007.
- Error-related electrocorticographic activity in humans during continuous movements.[J Neural Eng. 2012]Milekovic T, Ball T, Schulze-Bonhage A, Aertsen A, Mehring CJ Neural Eng. 2012 Apr; 9(2):026007.
- Differential representation of arm movement direction in relation to cortical anatomy and function.[J Neural Eng. 2009]Ball T, Schulze-Bonhage A, Aertsen A, Mehring CJ Neural Eng. 2009 Feb; 6(1):016006.
- High-frequency gamma activity (80-150Hz) is increased in human cortex during selective attention.[Clin Neurophysiol. 2008]Ray S, Niebur E, Hsiao SS, Sinai A, Crone NEClin Neurophysiol. 2008 Jan; 119(1):116-33.
- Quantifying auditory event-related responses in multichannel human intracranial recordings.[Front Comput Neurosci. 2010]Boatman-Reich D, Franaszczuk PJ, Korzeniewska A, Caffo B, Ritzl EK, Colwell S, Crone NEFront Comput Neurosci. 2010 Mar 19; 4():4.
- Review Task-related gamma-band dynamics from an intracerebral perspective: review and implications for surface EEG and MEG.[Hum Brain Mapp. 2009]Jerbi K, Ossandón T, Hamamé CM, Senova S, Dalal SS, Jung J, Minotti L, Bertrand O, Berthoz A, Kahane P, Lachaux JPHum Brain Mapp. 2009 Jun; 30(6):1758-71.
- Review Intracranial EEG and human brain mapping.[J Physiol Paris. 2003]Lachaux JP, Rudrauf D, Kahane PJ Physiol Paris. 2003 Jul-Nov; 97(4-6):613-28.
- Review Human gamma-frequency oscillations associated with attention and memory.[Trends Neurosci. 2007]Jensen O, Kaiser J, Lachaux JPTrends Neurosci. 2007 Jul; 30(7):317-24.
- Differential representation of arm movement direction in relation to cortical anatomy and function.[J Neural Eng. 2009]Ball T, Schulze-Bonhage A, Aertsen A, Mehring CJ Neural Eng. 2009 Feb; 6(1):016006.
- Human motor cortical activity recorded with Micro-ECoG electrodes, during individual finger movements.[Conf Proc IEEE Eng Med Biol Soc. 2009]Wang W, Degenhart AD, Collinger JL, Vinjamuri R, Sudre GP, Adelson PD, Holder DL, Leuthardt EC, Moran DW, Boninger ML, Schwartz AB, Crammond DJ, Tyler-Kabara EC, Weber DJConf Proc IEEE Eng Med Biol Soc. 2009; 2009():586-9.
- Quantifying auditory event-related responses in multichannel human intracranial recordings.[Front Comput Neurosci. 2010]Boatman-Reich D, Franaszczuk PJ, Korzeniewska A, Caffo B, Ritzl EK, Colwell S, Crone NEFront Comput Neurosci. 2010 Mar 19; 4():4.
- Comparison of seizure detection algorithms in continuously monitored pediatric patients.[J Clin Neurophysiol. 2007]Lee HC, van Drongelen W, McGee AB, Frim DM, Kohrman MHJ Clin Neurophysiol. 2007 Apr; 24(2):137-46.
- Detecting
epileptic seizures in long-term human EEG: a new approach to automatic
online and real-time detection and classification of polymorphic seizure
patterns.[J Clin Neurophysiol. 2008]Meier R, Dittrich H, Schulze-Bonhage A, Aertsen AJ Clin Neurophysiol. 2008 Jun; 25(3):119-31.
- A direct brain interface based on event-related potentials.[IEEE Trans Rehabil Eng. 2000]Levine SP, Huggins JE, BeMent SL, Kushwaha RK, Schuh LA, Rohde MM, Passaro EA, Ross DA, Elisevich KV, Smith BJIEEE Trans Rehabil Eng. 2000 Jun; 8(2):180-5.
- Unsupervised movement onset detection from EEG recorded during self-paced real hand movement.[Med Biol Eng Comput. 2010]Awwad Shiekh Hasan B, Gan JQMed Biol Eng Comput. 2010 Mar; 48(3):245-53.
- Free-paced high-performance brain-computer interfaces.[J Neural Eng. 2007]Achtman N, Afshar A, Santhanam G, Yu BM, Ryu SI, Shenoy KVJ Neural Eng. 2007 Sep; 4(3):336-47.
- Overt foot movement detection in one single Laplacian EEG derivation.[J Neurosci Methods. 2008]Solis-Escalante T, Müller-Putz G, Pfurtscheller GJ Neurosci Methods. 2008 Oct 30; 175(1):148-53.
- Unsupervised movement onset detection from EEG recorded during self-paced real hand movement.[Med Biol Eng Comput. 2010]Awwad Shiekh Hasan B, Gan JQMed Biol Eng Comput. 2010 Mar; 48(3):245-53.
- Brain control of movement execution onset using local field potentials in posterior parietal cortex.[J Neurosci. 2009]Hwang EJ, Andersen RAJ Neurosci. 2009 Nov 11; 29(45):14363-70.
- A direct brain interface based on event-related potentials.[IEEE Trans Rehabil Eng. 2000]Levine SP, Huggins JE, BeMent SL, Kushwaha RK, Schuh LA, Rohde MM, Passaro EA, Ross DA, Elisevich KV, Smith BJIEEE Trans Rehabil Eng. 2000 Jun; 8(2):180-5.
- Overt foot movement detection in one single Laplacian EEG derivation.[J Neurosci Methods. 2008]Solis-Escalante T, Müller-Putz G, Pfurtscheller GJ Neurosci Methods. 2008 Oct 30; 175(1):148-53.
- Free-paced high-performance brain-computer interfaces.[J Neural Eng. 2007]Achtman N, Afshar A, Santhanam G, Yu BM, Ryu SI, Shenoy KVJ Neural Eng. 2007 Sep; 4(3):336-47.
- Review Error detection mechanisms of the brain: background and prospects.[Int J Psychophysiol. 2005]Bechtereva NP, Shemyakina NV, Starchenko MG, Danko SG, Medvedev SVInt J Psychophysiol. 2005 Nov-Dec; 58(2-3):227-34.
- Review Neuropharmacology of performance monitoring.[Neurosci Biobehav Rev. 2009]Jocham G, Ullsperger MNeurosci Biobehav Rev. 2009 Jan; 33(1):48-60.
- Effects of crossmodal divided attention on late ERP components. II. Error processing in choice reaction tasks.[Electroencephalogr Clin Neurophysiol. 1991]Falkenstein M, Hohnsbein J, Hoormann J, Blanke LElectroencephalogr Clin Neurophysiol. 1991 Jun; 78(6):447-55.
- Modulation of activity in medial frontal and motor cortices during error observation.[Nat Neurosci. 2004]van Schie HT, Mars RB, Coles MG, Bekkering HNat Neurosci. 2004 May; 7(5):549-54.
- Error-related EEG potentials generated during simulated brain-computer interaction.[IEEE Trans Biomed Eng. 2008]Ferrez PW, del R Millan JIEEE Trans Biomed Eng. 2008 Mar; 55(3):923-9.
- Hierarchical error processing: different errors, different systems.[Brain Res. 2007]Krigolson OE, Holroyd CBBrain Res. 2007 Jun 25; 1155():70-80.
- Evidence for hierarchical error processing in the human brain.[Neuroscience. 2006]Krigolson OE, Holroyd CBNeuroscience. 2006; 137(1):13-7.
- Neural correlates of reach errors.[J Neurosci. 2005]Diedrichsen J, Hashambhoy Y, Rane T, Shadmehr RJ Neurosci. 2005 Oct 26; 25(43):9919-31.
- Error-related electrocorticographic activity in humans during continuous movements.[J Neural Eng. 2012]Milekovic T, Ball T, Schulze-Bonhage A, Aertsen A, Mehring CJ Neural Eng. 2012 Apr; 9(2):026007.
- Modulation of activity in medial frontal and motor cortices during error observation.[Nat Neurosci. 2004]van Schie HT, Mars RB, Coles MG, Bekkering HNat Neurosci. 2004 May; 7(5):549-54.
- Motor-cortical beta oscillations are modulated by correctness of observed action.[Neuroimage. 2008]Koelewijn T, van Schie HT, Bekkering H, Oostenveld R, Jensen ONeuroimage. 2008 Apr 1; 40(2):767-75.
- Separable neural mechanisms contribute to feedback processing in a rule-learning task.[Neuropsychologia. 2008]Zanolie K, Van Leijenhorst L, Rombouts SA, Crone EANeuropsychologia. 2008 Jan 15; 46(1):117-26.
- Different neurons in different regions of human temporal lobe distinguish correct from incorrect identification or memory.[Neuropsychologia. 2004]Ojemann GA, Schoenfield-McNeill J, Corina DNeuropsychologia. 2004; 42(10):1383-93.
- Cortical control of a prosthetic arm for self-feeding.[Nature. 2008]Velliste M, Perel S, Spalding MC, Whitford AS, Schwartz ABNature. 2008 Jun 19; 453(7198):1098-101.
- Neural control of computer cursor velocity by decoding motor cortical spiking activity in humans with tetraplegia.[J Neural Eng. 2008]Kim SP, Simeral JD, Hochberg LR, Donoghue JP, Black MJJ Neural Eng. 2008 Dec; 5(4):455-76.
- Learning to control a brain-machine interface for reaching and grasping by primates.[PLoS Biol. 2003]Carmena JM, Lebedev MA, Crist RE, O'Doherty JE, Santucci DM, Dimitrov DF, Patil PG, Henriquez CS, Nicolelis MAPLoS Biol. 2003 Nov; 1(2):E42.
- Neuronal ensemble control of prosthetic devices by a human with tetraplegia.[Nature. 2006]Hochberg LR, Serruya MD, Friehs GM, Mukand JA, Saleh M, Caplan AH, Branner A, Chen D, Penn RD, Donoghue JPNature. 2006 Jul 13; 442(7099):164-71.
- Instant neural control of a movement signal.[Nature. 2002]Serruya MD, Hatsopoulos NG, Paninski L, Fellows MR, Donoghue JPNature. 2002 Mar 14; 416(6877):141-2.
- Sensorimotor rhythm-based brain-computer interface (BCI): feature selection by regression improves performance.[IEEE Trans Neural Syst Rehabil Eng. 2005]McFarland DJ, Wolpaw JRIEEE Trans Neural Syst Rehabil Eng. 2005 Sep; 13(3):372-9.
- Error-related electrocorticographic activity in humans during continuous movements.[J Neural Eng. 2012]Milekovic T, Ball T, Schulze-Bonhage A, Aertsen A, Mehring CJ Neural Eng. 2012 Apr; 9(2):026007.
- Towards on-line adaptation of neuro-prostheses with neuronal evaluation signals.[Biol Cybern. 2006]Rotermund D, Ernst UA, Pawelzik KRBiol Cybern. 2006 Sep; 95(3):243-57.
- Unsupervised adaptation of brain-machine interface decoders.[Front Neurosci. 2012]Gürel T, Mehring CFront Neurosci. 2012; 6():164.
- Error-related electrocorticographic activity in humans during continuous movements.[J Neural Eng. 2012]Milekovic T, Ball T, Schulze-Bonhage A, Aertsen A, Mehring CJ Neural Eng. 2012 Apr; 9(2):026007.
- Response error correction--a demonstration of improved human-machine performance using real-time EEG monitoring.[IEEE Trans Neural Syst Rehabil Eng. 2003]Parra LC, Spence CD, Gerson AD, Sajda PIEEE Trans Neural Syst Rehabil Eng. 2003 Jun; 11(2):173-7.
- Online detection of error-related potentials boosts the performance of mental typewriters.[BMC Neurosci. 2012]Schmidt NM, Blankertz B, Treder MSBMC Neurosci. 2012 Feb 15; 13():19.
- Error-related EEG potentials generated during simulated brain-computer interaction.[IEEE Trans Biomed Eng. 2008]Ferrez PW, del R Millan JIEEE Trans Biomed Eng. 2008 Mar; 55(3):923-9.
- Towards a robust BCI: error potentials and online learning.[IEEE Trans Neural Syst Rehabil Eng. 2006]Buttfield A, Ferrez PW, Millán Jdel RIEEE Trans Neural Syst Rehabil Eng. 2006 Jun; 14(2):164-8.
- Coadaptive brain-machine interface via reinforcement learning.[IEEE Trans Biomed Eng. 2009]DiGiovanna J, Mahmoudi B, Fortes J, Principe JC, Sanchez JCIEEE Trans Biomed Eng. 2009 Jan; 56(1):54-64.
- Towards on-line adaptation of neuro-prostheses with neuronal evaluation signals.[Biol Cybern. 2006]Rotermund D, Ernst UA, Pawelzik KRBiol Cybern. 2006 Sep; 95(3):243-57.
- Unsupervised adaptation of brain-machine interface decoders.[Front Neurosci. 2012]Gürel T, Mehring CFront Neurosci. 2012; 6():164.
- Two-dimensional movement control using electrocorticographic signals in humans.[J Neural Eng. 2008]Schalk G, Miller KJ, Anderson NR, Wilson JA, Smyth MD, Ojemann JG, Moran DW, Wolpaw JR, Leuthardt ECJ Neural Eng. 2008 Mar; 5(1):75-84.
- Electroencephalographic (EEG) control of three-dimensional movement.[J Neural Eng. 2010]McFarland DJ, Sarnacki WA, Wolpaw JRJ Neural Eng. 2010 Jun; 7(3):036007.
- An online brain-machine interface using decoding of movement direction from the human electrocorticogram.[J Neural Eng. 2012]Milekovic T, Fischer J, Pistohl T, Ruescher J, Schulze-Bonhage A, Aertsen A, Rickert J, Ball T, Mehring CJ Neural Eng. 2012 Aug; 9(4):046003.
- Massively
Parallel Signal Processing using the Graphics Processing Unit for
Real-Time Brain-Computer Interface Feature Extraction.[Front Neuroeng. 2009]Wilson JA, Williams JCFront Neuroeng. 2009; 2():11.